Newsletter
Nicoleta Spînu, the vice-Chair of the Communication Working Group, interviews Iacer Calixto about where the natural language processing (NLP) and large language models (LLMs) stand at present and their role in healthcare. Iacer Calixto is an assistant professor of artificial intelligence (AI) in the Department of Medical Informatics at the Amsterdam UMC, University of Amsterdam. There, he investigates methods based on machine learning (ML) and NLP, including the incorporation of vision and world knowledge for problems in medicine and psychology.
What are NLP and LLMs and are these computational methods any different from machine learning and deep learning?
Artificial Intelligence or AI has to do with intelligent algorithms. In machine learning, a specific type of AI, we train a model to perform a task for which we need data to teach the model. Deep learning is machine learning with neural networks, roughly speaking. NLP uses machine learning for human languages. Most of the time, we are referring to written text and language, but we can also think of speech processing. Thus, NLP is part of AI and part of ML. It refers to the development of models to understand and generate language. By understanding a language, we mean to be able to read a text and make sense of it in a very broad sense in order to solve a task or recommend something, to make a prediction, or to establish a correlation. By generating language, we mean to generate an answer to a question, for example, or to generate a translation for a sentence. Large language models are the latest development in the NLP field. They are large neural networks with a specific model architecture that are trained on vast amounts of data. Until recently, this was raw text. Nowadays, most of them are running on proprietary knowledge, and we don’t know how much data were used. Some say that it can be in the order of magnitude of trillions of tokens. Also, LLMs can have many billions of parameters. Thus, LLMs are very large in terms of parameters and data they consume. This is roughly what GPT4 is - it can do very intelligent tasks for us, but at the same time, it still makes silly mistakes a child would not.
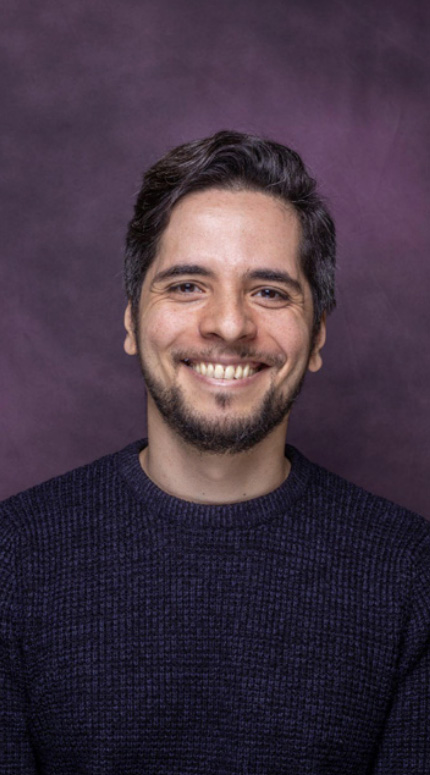
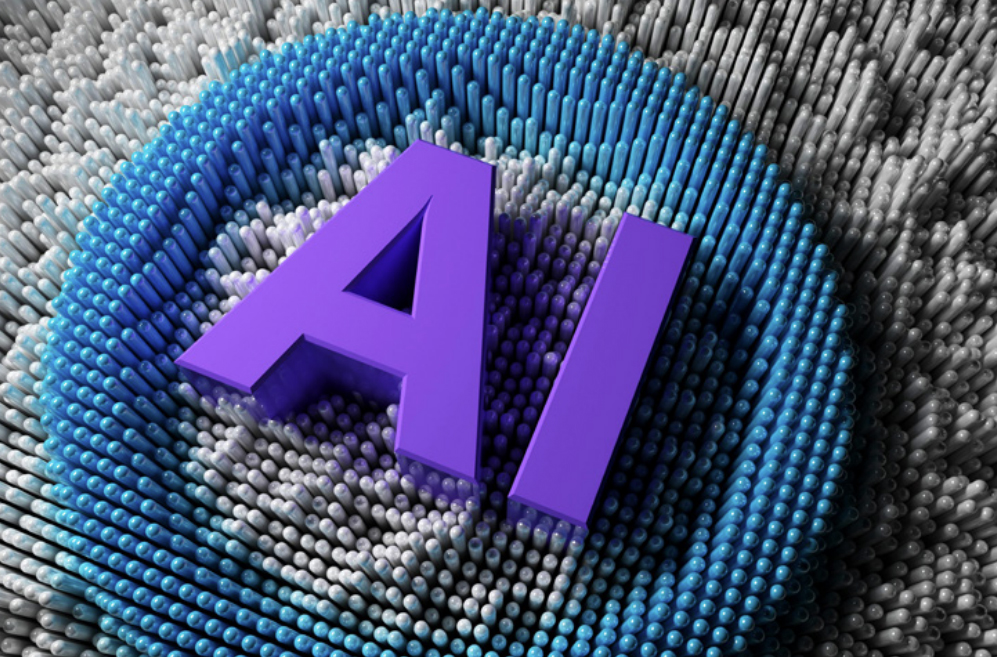
What kind of problems can NLP and LLMs be used for to solve in healthcare?
In the context of a hospital such as Amsterdam UMC, there are plenty of free texts available written by physicians, nurses, therapists, and other healthcare professionals. These free-text data, however, are messy and not good for clinical decision-making. One obvious task NLP can do is to structure such data into a FAIR format, i.e., Findability, Accessibility, Interoperability, and Reusability, which has to do with data quality. Every organization, especially in healthcare, strives to follow these principles, and NLP can be used to make sense of these messy free-text data and structure it FAIRly. In addition to that, NLP can be applied to any medical speciality, for example, in primary care, where general practitioners are used to writing notes. Another example is in radiology, generating reports from medical images to aid radiologists. Also, free-text data are available in Electronic Health Records (EHRs), and such data are useful in predicting the risk of patients for various conditions and diseases. One project we work on in our department focuses on the development of models to predict the risk for falls in elderly patients in the hospital. We employ NLP to test data to help clinicians identify patients at risk for falls. It is difficult to track the falling itself as it can be affected by different factors, such as medication. We also develop models to predict risk for cardiovascular disorders and different types of cancer. Lastly, NLP can be useful in patient phenotyping to identify patients with a specific condition or just before the onset of the condition.
What are the crucial aspects of the development of NLP and LLMs for real-world applications?
Development of NLP / LLMs models is a team effort. It requires synergy. Clinicians usually provide the question and the clinical context and relevance of the problem to be solved. A modeler might not actually know or easily identify problems such as the falling case I referred to above, which was for me. The biggest bottleneck remains to be the data. In healthcare, data are difficult to combine besides having access to high-quality data. For example, we don’t have enough data on rare diseases by design. When it comes to the NLP pipeline, you need to tailor it to the problem and there is still a need for modeling knowledge. Using LLMs, computational power becomes an issue but also, the interpretability of these models, understanding of what they do and how they provide the outcomes. It can be the case that a rather simple model, e.g., a logistic regression, can be of better use to help clinicians.
What are the biggest challenges in the development of NLPs and LLMs in healthcare at the moment?
One big issue remains to be the interpretability and explainability of such models. Nowadays, even by law, you are required to provide a certain level of transparency for a model to be considered fit for implementation. Another challenge is the validation of a model. High-quality data are hard to get access to and combine. The procedures take a very long time, e.g., up to a year, to have internal and external validation sets. Thus, access to data remains a big impediment and hinders advancements in healthcare research in general.
Could you tell us more about your research interests and projects you are investigating at present?
One research topic of my interest is synthetic data generation. We don’t have enough high-quality data, and getting access is very difficult for various reasons, including data protection regulations. In healthcare, everyone is protective. I believe in openness, and one way to solve this is by developing methods to generate synthetic patient data, including not only neat structured variables but also free text. Besides that, a project that I work on includes identifying patients with a high risk for different types of cancerbased on free text notes written by general practitioners in primary care using methods such as prompt-tuning. Another project involves the prediction of acute kidney injury in intensive care using longitudinal data and linking publicly available knowledge bases in partnership with a publishing company. In mental health, we develop NLP methods using social media data to flag the risk for specific mental health issues. These are models that can very easily allow you to forget information about a specific user if you need to.
What about your implications in the European Union-funded projects such as the IMAGINE and the Multi3Generation projects? What were the main outcomes? What kind of impact did such experiences have on your scientific career?
The IMAGINE project was an MSCA Global Fellowship, a personal grant to do my own research. It funded me for just under three years. I spent the time visiting New York University, where I worked on grounded language models (Calixto et al., 2021), vision and language (VL), and a novel benchmark designed for testing general-purpose pre-trained VL models (Parcalabescu et al., 2022).
Multi3Generation is a COST Action type of project where I was one of the initiators. It is a network of researchers who are interested in a certain topic, and it involves various European partners. My roles were short scientific mission leader, where I had the chance to oversee research visits and learn about what other colleagues work on. A survey on natural language generation conducted within the consortium is publicly available (Erdem et al., 2022). Currently, I am a Management Committee Member for the Netherlands.
Both the IMAGINE and Multi3Generation projects led to many fruitful collaborations, and some of those are still ongoing.
Any final thoughts to the MCAA members?
My recommendation is to stay away from the hype and choose to focus on what you do and do it well. On the other hand, as an academic, it is difficult to compete with tech companies head-to-head. If we want to train models, we don’t have that scale and capabilities. Choose wisely with whom to collaborate and the problems to work on.
Nicoleta Spînu
Postdoctoral researcher
Amsterdam UMC
hello@nicoletaspinu.com
Iacer Calixto
Assistant Professor
Department of Medical Informatics
Amsterdam UMC, University of Amsterdam
https://iacercalixto.github.io
linkedIn iacercalixto
References
Calixto I, Raganato A, Pasini T. (2021). Wikipedia Entities as Rendezvous across Languages: Grounding Multilingual Language Models by Predicting Wikipedia Hyperlinks. In Proceedings of the 2021 Conference of the North American Chapter of the Association for Computational Linguistics: Human Language Technologies, pages 3651–3661, Online. Association for Computational Linguistics.
Parcalabescu L, Cafagna M, Muradjan L, Frank A, Calixto I, Gatt A. (2022). VALSE: A Task-Independent Benchmark for Vision and Language Models Centered on Linguistic Phenomena. In Proceedings of the 60th Annual Meeting of the Association for Computational Linguistics (Volume 1: Long Papers), pages 8253–8280, Dublin, Ireland. Association for Computational Linguistics.
Erdem et al. (2022). Neural Natural Language Generation: A Survey on Multilinguality, Multimodality, Controllability and Learning. Journal of Artificial Intelligence Research 73: 1131-1207