Newsletter
I prompted ChatGPT to write an introduction about Large Language Models (LLMs) usage in medicine specifying it needed to be short and to sound like Nature Communications articles - the prompt: “Write a short introduction about LLMs usage in medicine in the style of a Nature Communication article.” The result is in the paragraph below.
Recent advances in artificial intelligence have led to the development of language models capable of processing vast amounts of medical data and generating accurate predictions and recommendations. These language models, known as large language models (LLMs), have the potential to revolutionize the field of medicine by improving diagnostic accuracy, enabling personalized treatment recommendations, and increasing access to medical expertise. However, their use in medicine is not without challenges, as there are concerns about potential biases, lack of transparency, and the risk of spreading misinformation.
Ornela Bardhi,
a personal account
Ornela Bardhi is a senior researcher and data scientist at Success Clinic, specializing in Real World Data from Nordic countries. Previously, Ornela worked as a scientific advisor to Albania's Minister of Health and Social Protection. She has a PhD in applied machine learning to medical data and is a founding member and the current Chairwoman of the board of the MCAA Western Balkans Chapter. She is passionate about science, technology, and innovation applied to the betterment of patient care, and the environment.
During April, I tested ChatGPT on some basic information. The results vary from funny and fake, to potentially useful. The first step was to establish the topic, test the chatbot with some basic terms and try to get some references. The other steps were more focused on refining the results.
I wanted ChatGPT to focus on LLMs in healthcare, specifically on diagnosis, self-diagnosis, and medications. During my MSCA PhD fellowship, I worked with cancer patients, and I know how difficult it is for them to receive the news of a cancer diagnosis. Most of the patients and their family and friends check the “doctor ‹insert search engine here›” about their disease and rely a lot on it, sometimes even more than their doctors. For this reason, it is imperative to discuss what such tools can and cannot do and how much we should trust them.
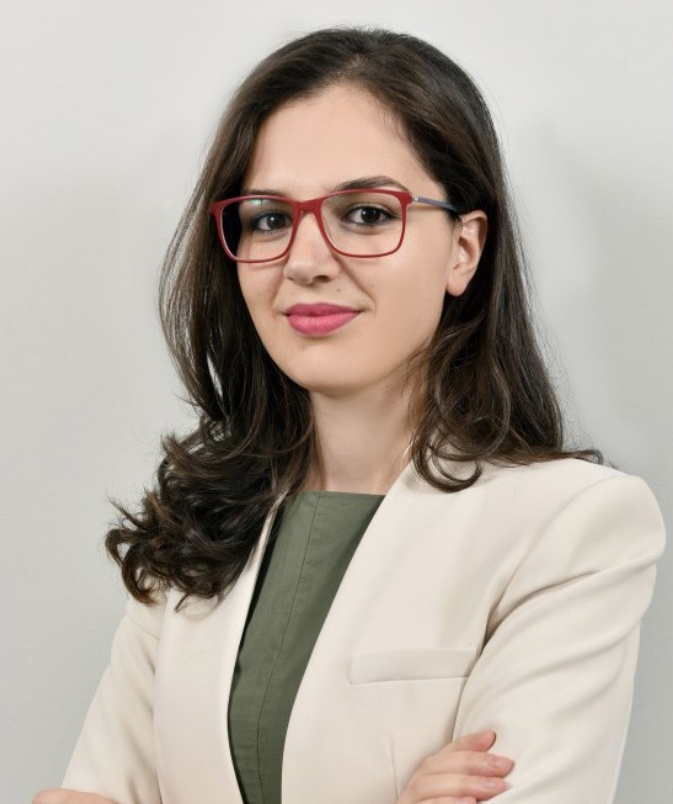
When I asked ChatGPT to write a short paragraph on how people have been using the internet and search engines to self-diagnose, it responded by writing that “LLMs have now substituted search engines and websites like WebMD, offering a more conversational symptom analysis. LLM-based self-diagnosis tools are now in use and have allowed people to quickly and easily identify potential medical issues, especially for those without access to medical professionals or who may be hesitant to seek medical advice.” It continued to mention that not everything is all roses, “there are concerns about accuracy and the risk of generating false positives or negatives,” and “despite these concerns, LLM-based self-diagnosis is likely to continue to grow in popularity, requiring improvements in accuracy and transparency, as well as educating the public on their limitations.”
According to ChatGPT, several companies have platforms and applications for self-diagnosis listing increased access to medical information and the potential for early detection of medical conditions as the main benefits. It also lists some risks, such as the potential for false positives (increased unnecessary tests and analysis) and false negatives (leading to delayed diagnosis and treatment), and lack of personalization to individual patients (lack of medical history).
When asked about LLMs usage on drug prescriptions, ChatGPT mentioned two examples, assisting with medication reconciliation in hospitals and developing personalized medication recommendations based on genetic information. It continues to write that LLMs can analyze large datasets of medical records and genetic information to provide accurate and personalized medication recommendations. It cites a study published in the Journal of the American Medical Informatics Association where an LLM-based model predicted a patient's medication regimen with an accuracy of over 90%. I was curious and wanted to learn more about the study, so I asked for the title and authors of the article. To this day, I still cannot find the article because it does not exist.
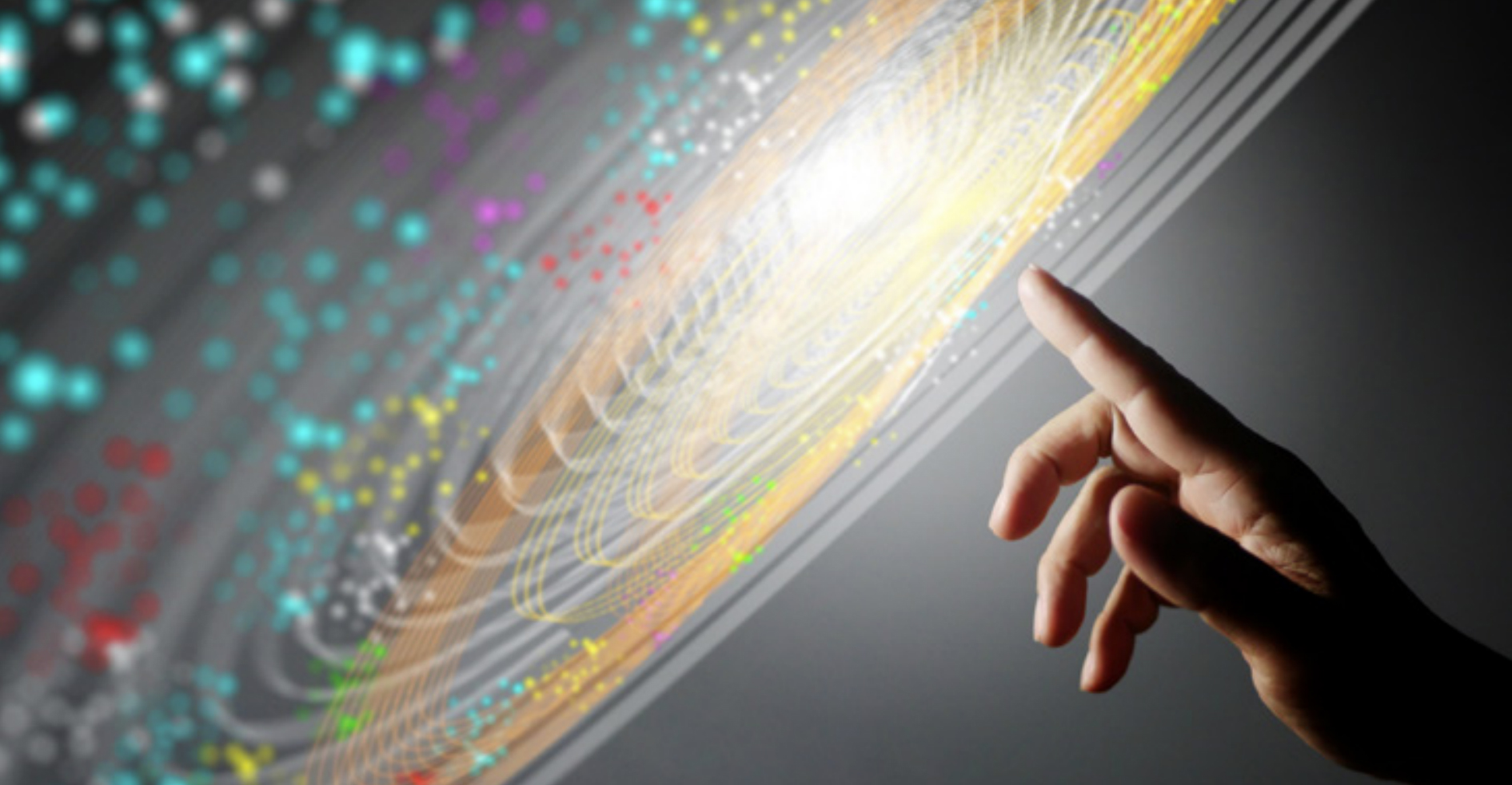
Such a phenomenon is called “model hallucination” (some researchers are opposed to the term and prefer using “prediction error”), and it is not something new with LLMs. Since 2022, many scholars and users of any AI-powered chatbot have encountered confident responses not supported by the input data or are otherwise implausible, which can be particularly dangerous in medical settings where decisions can have life-ordeath consequences. An LLM-based tool might generate a diagnosis or treatment recommendation that is not supported by the patient's medical history or test results but is instead based on patterns that the LLM has identified in the training data.
I often work with Anatomical Therapeutic Chemical (ATC) codes. The codes are easy to find and are publicly available from the World Health Organization (WHO) website. I wanted to test if I could get the information from ChatGPT. I thought this would be straightforward and I would not encounter any mistakes. Well, I was wrong. ChatGPT decided to invent some new compounds and ATC codes or mix the ATC codes of different drugs.
Upon further questioning and probing, ChatGPT mentions some other drawbacks associated with the use of LLMs in medicine, including:
• Bias: LLMs are trained on medical data biased towards a demographic or geographic location, and the responses may not apply to other populations leading to the perpetuation of incorrect medical information or diagnoses;
• Lack of transparency: LLMs are often described as "black boxes" because the process by which they arrive at their predictions is not easily understandable by humans. This lack of transparency can make it difficult for medical professionals to trust and effectively utilize LLM-based tools;
• Ethical concerns: LLMs can raise ethical concerns around patient privacy and data security (data collection, storage, and usage);
• Overreliance on technology: While LLMs can be a valuable tool in medical decision-making, there is a risk that medical professionals may become over-reliant on technology and neglect to consider other important factors, such as patient history and context;
• Cost: The development and implementation of LLM-based tools can be expensive, which may limit their access to certain healthcare systems or patient populations;
Legal and regulatory challenges: As LLMbased tools become more widely used in medicine, there are likely to be legal and regulatory challenges around issues such as liability, safety, and accuracy.
In general, when using ChatGPT or similar LLMs, one must remember that these models are trained on an enormous amount of internet data. Part of that data is factual, fair, and harmless, and the other is misinformed, biased, and harmful material. LLMs are probabilistic algorithms, so if you prompt the same question multiple times, you might get some variations of the same answer or sometimes even a different one. While they are fascinating and, at times, helpful (depending on the use case), the technology has a lot to improve; however, I do not think it will take 50 years to do so.
Ornela Bardhi
Senior researcher, Success Clinic
ornela.bardhi@successclinic.fi
Twitter @ornelabardhi