Newsletter
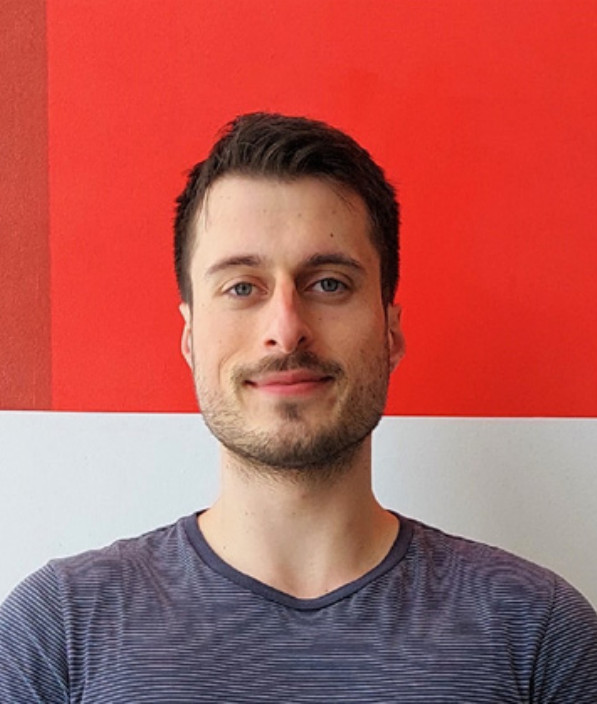
The rise of Artificial Intelligence (AI) is revolutionizing scientific research, offering new capabilities in data analysis and predictive modeling. However, this technological marvel comes with significant environmental costs due to its heavy reliance on energy and specialized hardware. As AI becomes integral to research, balancing its benefits with sustainability practices is crucial to minimizing its ecological footprint.
Bionote
Quentin Loisel is a current MSCA PhD researcher at Glasgow Caledonian University, UK, and the MCAA UK Chapter Chair. He is part of the Health CASCADE project, which aims to make co-creation trustworthy. He is developing technologies for this complex collective intelligence, democratic, and evidence-based process. His work bridges technology and the fundamental human dimension within the values of the co-creation process. With the recent rise of generative AI, he actively explores its potential for cognition, collaboration, and science. With technology's growing influence in our society, Quentin aims to enable collaboration between society’s actors to make the most of future technology.
Artificial Intelligence (AI) is transforming the landscape of scientific research, offering unprecedented capabilities in data analysis, predictive modeling, augmentation, and automation. Over the past decade, it has become integral to some scientific methodologies, leading to groundbreaking discoveries. For instance, AI has enabled researchers to predict protein structures with remarkable precision (Schauperl & Denny, 2022) and to model complex climate scenarios that were previously infeasible (Jones et al., 2023). Generative AI technologies' opening and fast development have passed a new milestone. Characterized by their accessibility and versatility, specialized AI models can relevantly interact with the language and support all the steps of the research workflow across any field. Their adoption could increase productivity while improving the quality and accessibility of science. These qualities push to an ever-increasing use and potential general adoption. However, a significant environmental toll lies beneath these technological marvels.
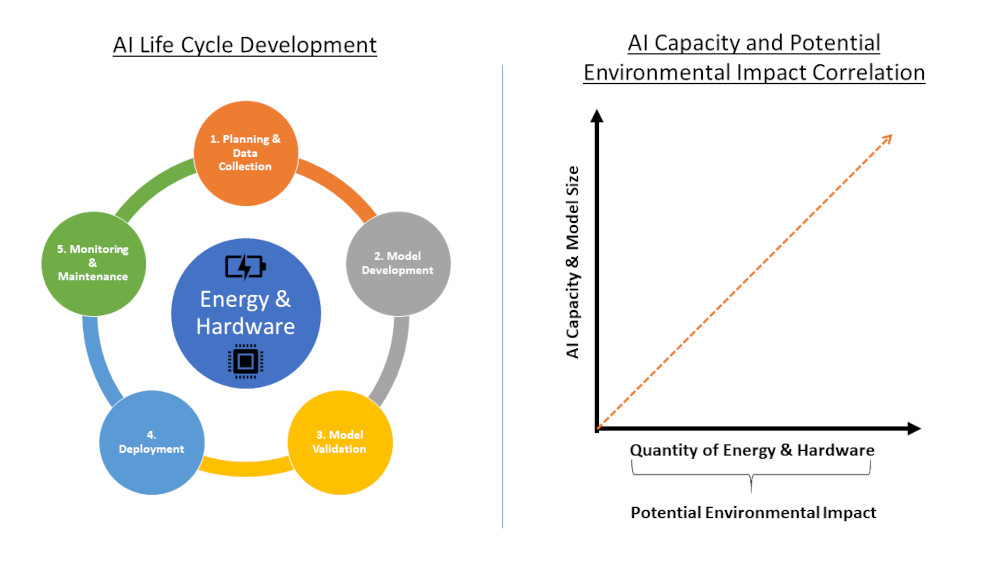
The environmental costs of AI
The AI life cycle implies five stages: 1) planning and data collection, 2) model development, 3) mode validation, 4) deployment, and finally, 5) monitoring and maintenance. This cycle requires two primary resources: hardware and energy. Indeed, AI technologies rely heavily on specialized hardware, such as graphics processing units (GPUs) and data centers, which require rare and precious materials. The extraction of these materials often involves environmentally damaging mining practices.
Moreover, training AI models can be an energyintensive process; one study estimated that training some AI models can generate as much carbon dioxide as five cars over their lifetimes (Luo et al., 2023). This high energy consumption contributes to carbon emissions and strains our energy resources.
Compared to other technological advancements, AI's environmental impact is particularly stark. For instance, traditional computing systems, while energy-consuming, do not match the intensity of AI’s resource demands. However, not all AI models have the same environmental impact. A small model could be trained on your computer in a few hours, using your existing hardware and consuming only a few watts. However, until now, the model size has been a central factor of AI progression capacity. This can be seen in the name of the promising “Large Language Models” (e.g., ChatGPT), where “Large” marks the distinction from the previous smaller “Language Models.” The larger a model is, the more data, computing power, and energy you need. In short, AI capacity, model size, necessary infrastructure, energy, and environmental impact are positively correlated. Generative AI models confirm this tendency, with bigger models showing more versatile capacity. Couple this correlation with a potential generalization of AI in science, and you will understand how all science could exponentially carbonate.
Toward sustainable AI in science
The AI industry works to develop and optimize energy needs and hardware efficiency to save costs during the development cycle. In this situation, we can speak about research for AI sustainability. However, waiting for technological advances to solve environmental impact is not a good strategy. Instead, it is about engaging the research ecosystem in sustainable practices. As the scientific community embraces AI, research actors must consider a new range of questions, from ethical usages to deploying and securing infrastructures. This is a timely opportunity to imply sustainability in these questions and ensure the evolution is made accordingly. Another good point is that we do not start from scratch; we already have the material to consider sustainable technology deployment (Mohamed Hashim et al., 2022) and research practices (Ligozat et al., 2020).
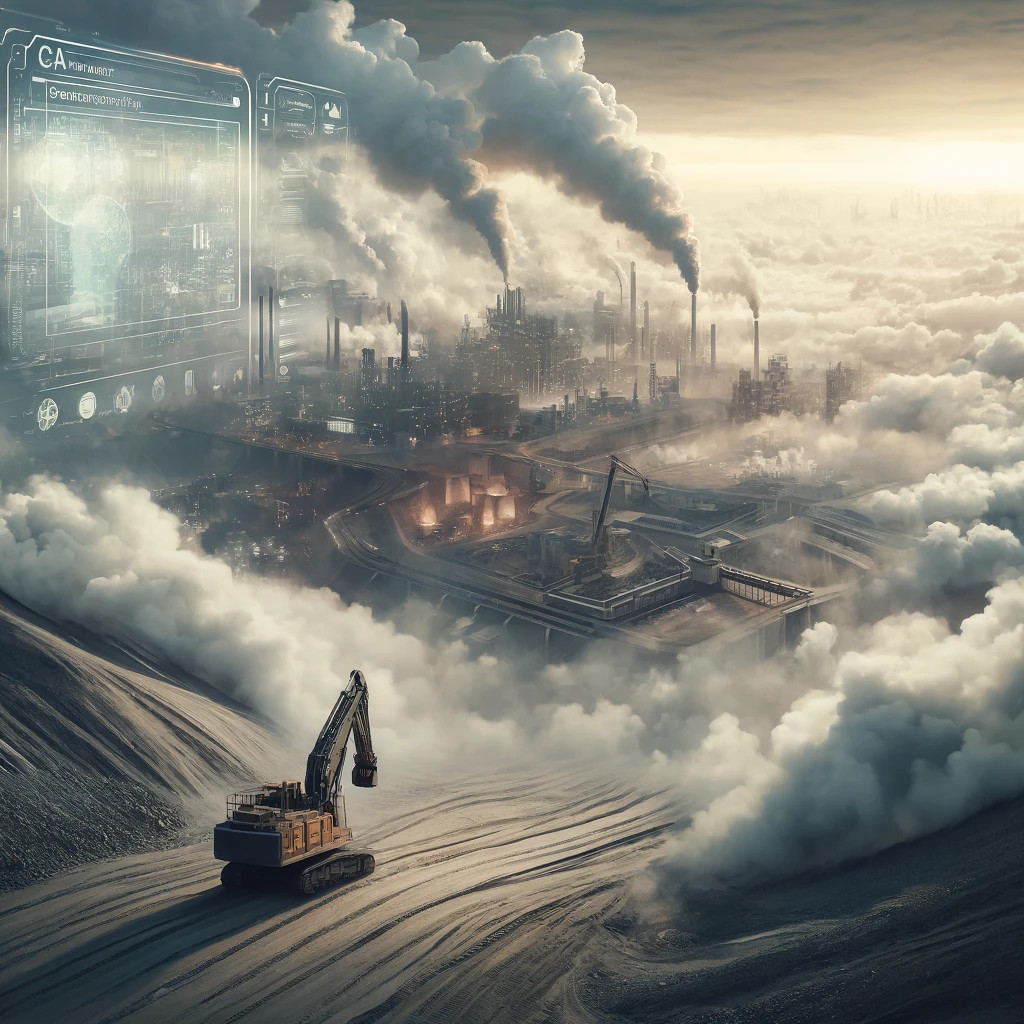
However, what does not consider these resources is the potential general adoption of generative AI models. For research institutions, this means making AI technologies available to their communities and making decisions toward efficient data management, green data centers, recycling electronic devices, energy-saving hardware, virtualization, cloud computing, sustainable deployment practices, and continuous system optimization. These are crucial for reducing the environmental impact of AI technologies. Specifically, using open-source models and developing narrow tools based on smaller but specialized models would avoid training unnecessary additional models. Hardware lifecycle management and organizational commitment to sustainability policies would further enhance this effort.
Best practices for AI needed
Indeed, like most other digital technologies, the environmental impact of using these AI tools is hidden, and their accessibility calls users for trivial usage. For example, the current working guidelines on using generative AI for research (European Commission, 2024) don’t include an environmental dimension. A potential reason is that these guidelines still struggle to establish the appropriate use of these technologies. However, this would be necessary to evaluate the ecological impact of generative AI in science. This is a new field for metascience, and answering this question would allow the development of best practices for optimal usage in science and its environmental impact.
This question becomes urgent since the adoption and the environmental impact of research with it are growing. As we continue to harness AI’s power, we must ensure its ecological footprint does not overshadow its scientific benefits as it evolves.
Quentin Loisel
MSCA PhD candidate
Glasgow Caledonian University
quentin.loisel@gcu.ac.uk
References:
European Commission. (2024). Living guidelines on the responsible use of generative AI in research. https://research-and-innovation.ec.europa.eu/document/2b6cf7e5-36ac-41cb-aab5- 0d32050143dc_en
Jones, A., Kuehnert, J., Fraccaro, P., Meuriot, O., Ishikawa, T., Edwards, B., Stoyanov, N., Remy, S. L., Weldemariam, K., & Assefa, S. (2023). AI for climate impacts: Applications in flood risk. Npj Climate and Atmospheric Science, 6(1), 1–8.
Ligozat, A.-L., Névéol, A., Daly, B., & Frenoux, E. (2020). Ten simple rules to make your research more sustainable. PLoS Computational Biology, 16(9), e1008148.
Luo, T., Wong, W.-F., Goh, R. S. M., Do, A. T., Chen, Z., Li, H., Jiang, W., & Yau, W. (2023). Achieving green AI with energy-efficient deep learning using neuromorphic computing. Communications of the ACM, 66(7), 52–57.
Mohamed Hashim, M. A., Tlemsani, I., & Duncan Matthews, R. (2022). A sustainable university: Digital transformation and beyond. Education and Information Technologies, 27(7), 8961–8996.
Schauperl, M., & Denny, R. A. (2022). AI-based protein structure prediction in drug discovery: Impacts and challenges. Journal of Chemical Information and Modeling, 62(13), 3142–3156.